What is Nonresponse Bias
A Comprehensive Guide to Understanding and Avoiding It
In this article
- What is Nonresponse Bias?
- Why is it Important?
- Causes of Nonresponse Bias in Online Polls
- Strategies to Mitigate Nonresponse Bias
- Identifying and Analyzing Nonresponse Bias
- Tools for Mitigating Nonresponse Bias
- Real-World Examples
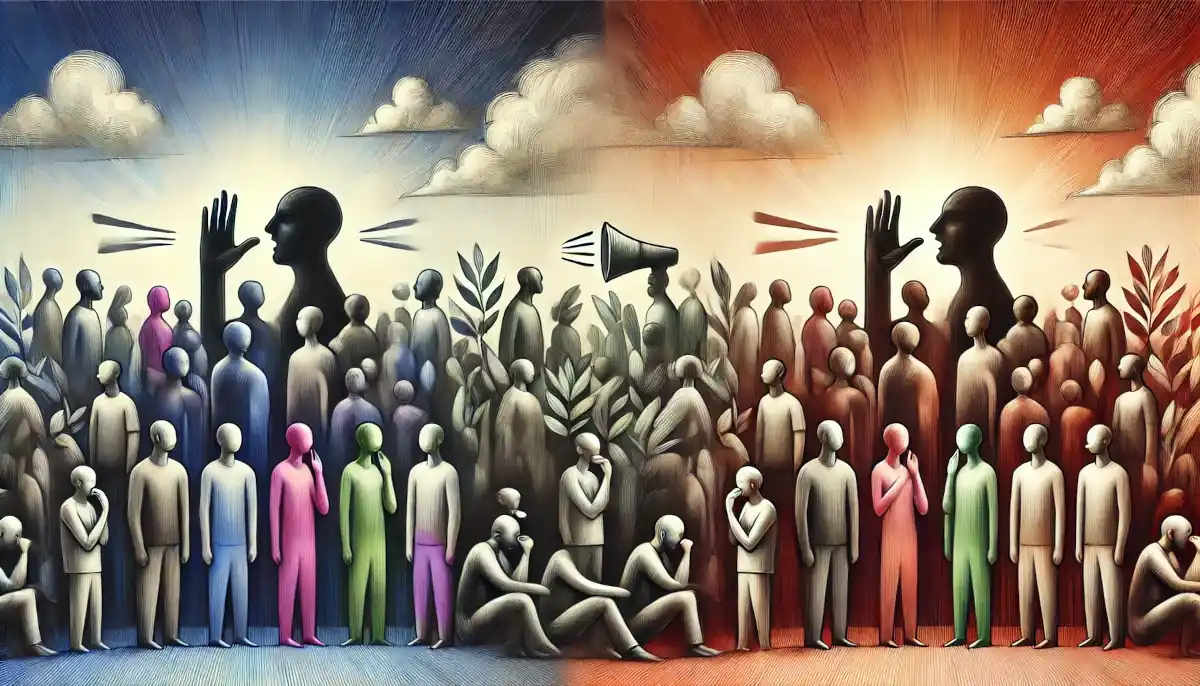
What is Nonresponse Bias?
Nonresponse bias is a significant challenge in online polling that can drastically affect the validity and reliability of your results. This type of bias arises when the individuals who do not participate in a poll differ systematically from those who do. These differences can lead to skewed data, as the results may not accurately reflect the views of the broader population. For example, if a particular demographic group is less likely to respond, their perspectives may be underrepresented, leading to misleading conclusions.
The impact of nonresponse bias is particularly pronounced when response rates are low, often becoming a critical issue when participation drops below 70%. However, even with higher response rates, nonresponse bias can still occur and distort the findings. To minimize this bias, it's essential to compare the characteristics of respondents with those who did not respond, ensuring that the sample is as representative as possible.
In this guide, we'll explore the intricacies of nonresponse bias in the context of online polls, why it matters, and practical strategies to mitigate its effects, ensuring your poll results are accurate and reliable.
Why is it Important?
Nonresponse bias can significantly undermine the validity of your online polls, making it a critical issue to address. Here's why it's important to understand and mitigate its effects:
- Inaccurate Representation:
When a significant portion of your target audience does not respond to a poll, the views of those who do respond may not accurately reflect the broader population. This can lead to an unbalanced dataset where certain demographics, opinions, or behaviors are underrepresented or completely absent. For example, if younger individuals or those with lower incomes are less likely to participate in your poll, the results will skew towards the perspectives of those who did respond, often those who are older or more affluent. This lack of representation can lead to data that is not just incomplete but also misleading, particularly if important segments of your audience are systematically excluded.
- Misleading Conclusions:
Decisions made based on biased data can have far-reaching consequences. If your online poll results are skewed due to nonresponse bias, any conclusions drawn from this data may be flawed. For instance, a business making strategic decisions based on an online poll that underrepresents a key customer segment might develop products or services that do not meet the needs of their entire market. In public opinion polling, this can lead to incorrect forecasts or policy recommendations that fail to address the needs or concerns of the general population. Ultimately, misleading conclusions can result in strategies that are ineffective at best, and harmful at worst, potentially leading to wasted resources and missed opportunities.
- Erosion of Credibility:
In any research or data-driven endeavor, credibility is paramount. If stakeholders - whether they be clients, colleagues, or the public - begin to suspect that your data is biased, it can severely damage your reputation. Nonresponse bias, when not properly addressed, can lead to a loss of trust in the integrity of your research. For organizations that rely on accurate data to inform decisions, this can have long-term implications, as stakeholders may become skeptical of future polls or studies. Furthermore, in fields such as market research, public policy, or journalism, maintaining credibility is essential for ensuring that your findings are taken seriously and acted upon.
Causes of Nonresponse Bias in Online Polls
Nonresponse bias in online polls can stem from various factors, including:
- Poll Design: Lengthy or complex polls can deter responses.
- Distribution Channels: Polls distributed only through certain channels, like social media or email, may exclude individuals not active on those platforms. Learn more about different distribution strategies in our Guide on How to Make Polls.
- Time of Polling: The timing of when the poll is administered can affect who is available to respond.
- Perceived Relevance: If respondents do not see the poll as relevant to them, they are less likely to participate.
How to Avoid Nonresponse Bias
Nonresponse bias is a challenge that can't be completely eliminated, but there are several effective strategies you can implement to minimize its impact and ensure your online poll data is as representative as possible:
- Improve Poll Design:
- Keep it Concise: Shorter polls generally have higher response rates because they require less time and effort to complete. Learn more about survey length and response rates.
- Simplify Language: Avoid using jargon or complex language that could confuse respondents. Clear and simple wording encourages broader participation, especially from diverse demographic groups. See common survey question mistakes.
- Logical Flow: Ensure that your poll follows a logical sequence of poll questions that maintains the respondent's engagement throughout the process.
- Incentivize Participation: Offering incentives, such as digital rewards, discounts, or entry into a prize draw, can motivate more people to complete your online poll. Be sure the incentive is appropriate for your audience and doesn't introduce bias. Read about the impact of incentives on survey participation.
- Diversify Polling Channels: Use multiple channels to reach your audience, such as email, social media, and website pop-ups. This approach helps capture responses from a diverse group, not just those who are highly engaged on one platform.
- Follow-Up Reminders: Sending polite reminders to those who have not yet completed the poll can significantly boost response rates. However, it's important to time these reminders carefully to avoid being perceived as spam.
- Weighting Responses: When nonresponse bias is unavoidable, applying statistical weighting to your responses can help correct the imbalance and provide a more accurate reflection of the target population. This technique adjusts the data to better represent underrepresented groups. Understanding response weighting methods.
By implementing these strategies, you can greatly reduce the impact of nonresponse bias on your online polls, ensuring that your results are both accurate and reliable. Additionally, it's important to continuously evaluate and refine your polling methods to keep up with changes in respondent behavior and technological advancements.
Identifying and Analyzing Nonresponse Bias
Detecting nonresponse bias in online polls requires careful analysis. Here are some methods to identify and assess its impact:
- Compare Respondents vs. Nonrespondents: Examine demographic and behavioral differences between those who responded to the poll and those who did not. Significant differences can indicate the presence of nonresponse bias.
- Conduct Follow-Up Polls: A follow-up poll targeting nonrespondents can provide insights into why they chose not to participate and whether their views differ from those who did respond.
- Use Statistical Tests: Employ statistical techniques such as Chi-square tests or logistic regression to determine if nonresponse is random or systematic. This can help you understand the extent of the bias.
Tools for Detecting and Mitigating Nonresponse Bias
Several tools can assist in identifying and mitigating nonresponse bias in online polls. Below are some that are commonly used in polling research:
- R: A programming language and software environment for statistical computing. R offers packages like
survey
that are tailored for poll analysis. - Stata: A comprehensive statistical software that includes modules for poll analysis and adjustments for nonresponse bias.
- SPSS: Another popular statistical analysis tool that offers robust capabilities for dealing with nonresponse bias.
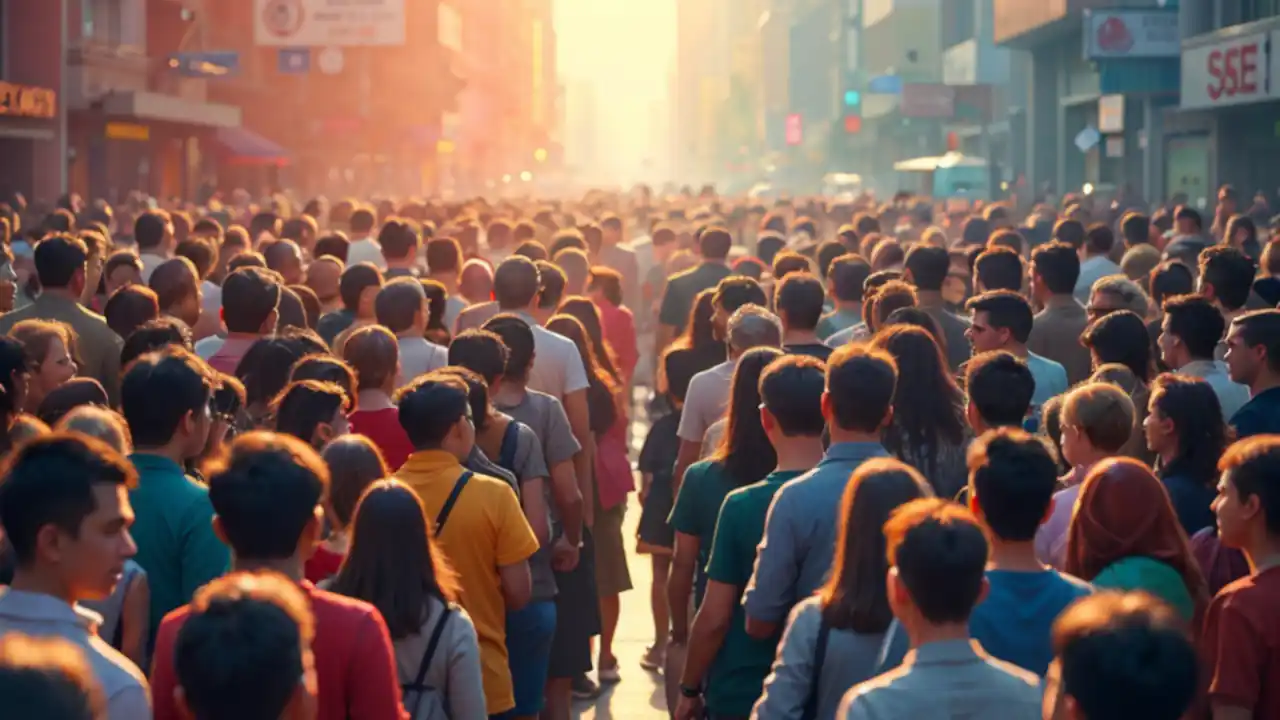
Real-World Examples of Nonresponse Bias
To further illustrate the impact of nonresponse bias, let's explore two detailed case studies that highlight what went wrong and how it could have been avoided:
- Political Online Polling:
A well-known example of nonresponse bias in political polling occurred during the 2016 U.S. Presidential Election. Many polls predicted a victory for Hillary Clinton, but they failed to accurately capture the support for Donald Trump. One of the key issues was nonresponse bias, particularly among rural and working-class voters who were less likely to participate in online and telephone polls. These groups were underrepresented in the sample, leading to a skewed perception of candidate popularity.
What went wrong: Pollsters relied heavily on traditional polling methods that failed to engage certain voter demographics. The nonresponse bias was exacerbated by the fact that these nonresponding groups had different voting intentions than those who participated.
How it could have been avoided: To reduce nonresponse bias, pollsters could have employed a more diverse set of polling methods, including reaching out to voters via channels they were more likely to engage with, such as in-person interviews in rural areas or mobile-friendly online polls. Additionally, adjusting the weighting of responses to account for underrepresented demographics could have provided a more accurate picture.
- Consumer Preference Polls:
In 2014, a major consumer electronics company conducted an online poll to gauge customer preferences for a new product line. The poll results indicated a strong preference for high-end, feature-rich models, leading the company to focus its marketing efforts on these products. However, when the products were released, sales were disappointing. Further analysis revealed that the poll had been biased towards tech-savvy respondents who were more likely to favor advanced features, while casual users who preferred simpler, more affordable models were underrepresented.
What went wrong: The online poll was distributed primarily through tech forums and social media channels frequented by enthusiasts, leading to a nonresponse bias where the preferences of the general consumer base were not accurately captured.
How it could have been avoided: The company could have diversified its polling channels to reach a broader audience, including casual users who may not frequent tech forums. Offering incentives for participation across different demographic groups and ensuring the poll was easily accessible on multiple platforms (e.g., email, mobile, in-store) could have resulted in a more balanced sample.